- Understanding AI
- What is Predictive AI?
- How does Predictive AI work?
- What is Generative AI?
- How does Generative AI work?
- What’s the difference? Should you choose a favorite?
- Real-world applications of Predictive AI
- Use of Generative AI in Business Operations
- Making the Final Call: Predictive AI vs Generative AI
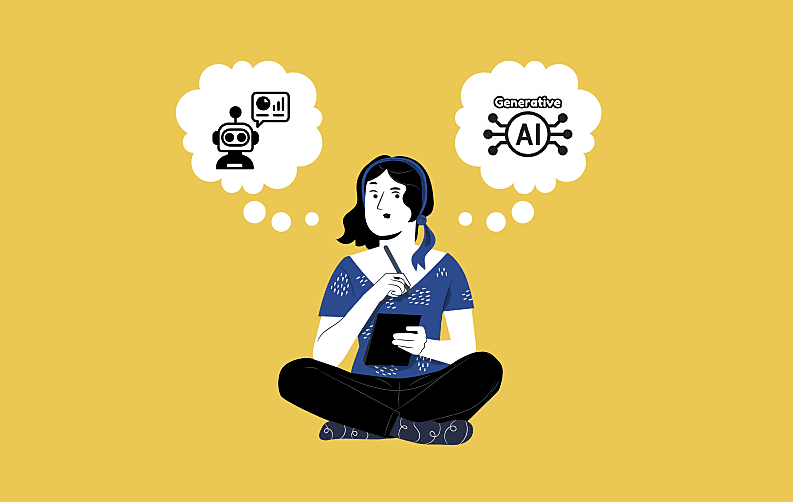
Ever since Sam Altman’s led company OpenAI introduced AI tools like ChatGPT and Dal-E, the entire tech and business landscape has witnessed a foundational shift. These tools have given birth to a new Gold Rush attracting eyeballs from all around the globe.
But this fascination for AI/ML is not without reason. These tools enable businesses to reap AI and ML benefits to supercharge their business performance. And with the popularity of AI going through the roof, different subsets like generative AI and Predictive AI are also gaining a lot of traction.
Generative AI and predictive AI are two largely known branches of Artificial Intelligence that are now commonly used in the real world. Once amalgamated within mobile applications and other software, these technologies can deliver unprecedented customer service and personalization.
However, like Machine Learning and Deep Learning, these technologies are so tangled that laymen often fail to see the distinction. But all hope is not lost! Today, we will explain the intricacies of generative AI vs Predictive AI that will help you end this ongoing debate.
So, let's jump on board the bandwagon and dive into the realm of artificial intelligence and data-led outputs.
Understanding AI
Before entering the facade of generative AI vs Predictive AI, it's crucial to understand what AI actually is. Though AI is giving us a glimpse into the future, it is not what we have seen in the movies (no robot will come from the future for Sarah Connor). The history of AI rolls back to the ages, and versions of it can be seen throughout cultures, regions, and even mythologies.
AI or artificial intelligence is simply the science and technology that aims to create intelligent machines that can think, learn, and solve problems like humans. It's like building a computer or software that can mimic human intelligence more precisely and efficiently.
AI enables machines to perform tasks that typically require human intelligence, such as understanding natural language, recognizing images, playing games, making predictions, and much more. It uses complex algorithms and data analysis to learn from examples and experiences, allowing the AI system to improve its performance over time.
Artificial Intelligence act as intelligent machines that can learn and perform tasks while bringing greater automation and intelligence to our modern world. These advancements include virtual assistants like Siri and Alexa, self-driving cars, and automated robots to foster convenience and even save lives.
What is Predictive AI?
As we said before, Predictive AI is a subset of artificial intelligence that leverages the powers of advanced algorithms, statistical models, and machine-learning techniques to forecast future trends, behaviors, or outcomes.
Unlike traditional AI, which focuses on processing data to perform specific tasks, Predictive AI takes it up a notch by going beyond the present and forecasting future outcomes. This data could encompass various topics - from past customer interactions to stock market performances or intricate medical records.
If fed accurate and reliable data into the system, Predictive AI can analyze these datasets, detect data flow anomalies, and infer how they will play out regarding results or behavior.
Moreover, Predictive AI adds another dimension and greater accuracy to solutions, ultimately increasing the chance of success and achieving positive business outcomes. The technology facilitates data-driven decision-making regarding strategy development. Thus, improving overall efficiency, profit margins, and enhanced customer satisfaction levels.
How does Predictive AI work?
The captivating abilities of Predictive AI originate from its intricate working mechanism. At its core, Predictive AI uses systematic data collection and processing techniques to make educated guesses about what might happen next. Here's a simplified overview of the backend of predictive AI:
1. Data Collection
Predictive AI relies heavily on data like any other AI model. Predictive AI consumes humongous pools of historical data related to the subject of interest. Predictive AI diligently handles these issues and lays a solid foundation for accurate forecasting. For instance, this data might infer customer behaviors, past sales figures, market trends, or medical records. This raw data is then collected and organized in a data warehouse or lake.
2. Data Preprocessing
Unprocessed or raw data is like crude oil; it doesn't hold much value until processed and filtered. Unstructured datasets often contain noise, errors, or missing values, which means they will not generate any reliable value until these adulterations are taken care of.
Predictive analytics comes into play here and performs a thorough cleaning and processing of these raw datasets, ensuring it's accurate and consistent to generate reliable results.
3. Selecting algorithms
Just collecting and processing data will not cut it; selecting an algorithm favorable to your goals is just as important. Almost every AI model relies heavily on algorithms to assess patterns and pump out results. Choosing the right algorithm is more than crucial, as the result can only be as accurate as the algorithm's level of accuracy.
Some majorly used ML algorithms are:
- Linear regression,
- Searching Algorithms,
- Graph Algorithms
- Decision trees,
- Computational Geometry Algorithms,
- Neural networks
4. Feature training
After identifying the ideal algorithm, the next step is to train it on specific features the model will detect. Predictive AI employs machine learning algorithms, such as linear regression, decision trees, and neural networks, to train its predictive models. These features act as the building blocks for predicting future outcomes.
For example, If we predict customer churn for a telecom company, relevant features might include call duration, customer tenure, and service usage patterns. Training your algorithm on such feature selection is critical as it directly affects the predictive model's performance.
5. Prediction and model evaluation
Once the models are trained and polished, the only thing remains to use the model to make predictions. Now, fresh data unseen during the training phase is fed into the trained models.
By feeding new data into these models, they can make educated guesses about future outcomes with impressive accuracy. Then, the model evaluates the data by analyzing the knowledge gained from the training phase and generates predictions about future results.
These predictions can be numerical values (stock prices or weather temperature) or binary classifications (whether a customer will purchase a product).
What is Generative AI?
Unlike predictive AI, Generative AI is generally used to create new content, including audio, code, images, text, simulations, and videos.
Generative AI takes a different approach, creating new and original content. It employs sophisticated algorithms to generate novel outputs that mimic human-like creativity. By learning from large datasets, generative AI models can generate text, images, music, and even videos that exhibit high authenticity.
For many years, generative models faced challenging tasks, such as learning to create photorealistic images or providing accurate textual information in response to questions. Progress in this area was slow as these tasks were highly complex. Meaning the technology of that time did not have sufficient bandwidth to support the computation requirements.
However, as the computation power increased, it enabled machine learning (ML) teams to build foundation models: Massive unsupervised models that train ridiculous amounts of data. With accelerated computation, ML engineers have now calibrated these generative foundation models capable of generating almost indistinguishable outputs from humans.
How does Generative AI work?
Generative AI models infused with neural networks have the remarkable ability to learn from existing data. It allows them to generate new, original, catchy, and human-like content. A significant advancement in generative AI comes from diverse learning approaches like unsupervised or semi-supervised learning during training.
These approaches enable organizations to efficiently leverage vast amounts of unlabeled data efficiently, laying the groundwork for foundational models. These foundational models act as a strong basis for AI systems capable of performing various tasks.
Typically, Generative AI uses three prominent techniques:
- Variational Autoencoders (VAEs)
- Generative Adversarial Networks (GANs)
- Denoising Diffusion Models
Generative AI Interfaces
GPT-3 and Stable Diffusion are today the primary examples of generative AI. These platforms are at the forefront of AI revolutions and have propelled language-related applications. For instance, ChatGPT, built upon GPT-3, allows users to generate essays based on short text requests. Meanwhile, Stable Diffusion enables the generation of photorealistic images from text input. The image below illustrates the three essential requirements for a successful Generative AI model.
What’s the difference? Should you choose a favorite?
By now, you’re probably aware that while both technologies share a technology pallet, comparing them is like comparing apples to oranges. Top artificial intelligence companies know that these two fruits from the same tree can benefit companies in their own way. Generative AI and Predictive AI are different types of artificial intelligence with distinct functionalities. The main difference between predictive and generative AI lies in their core functionalities.
While both generative and predictive subsets of Artificial Intelligence use machine learning algorithms, generative AI generates new content based on learned patterns, while Predictive AI analyzes existing data to make predictions.
Predictive AI is the go-to choice for tasks that require forecasting or decision-making. While Generative AI, on the other hand, is largely preferred in creative efforts when there is a need to create new content.
Moreover, predictive AI vs generative AI learning is different. Generative systems tend to be less interpretable than those relying purely on statistics or machine learning. Due to their usage of latent space representations, which can encode said features into abstract concepts not immediately recognizable by humans yet still produce efficient results better than traditional narrower ones.
Aspect | Predictive AI | Generative AI |
Objective | Predict future outcomes based on historical data | Generate new and original content |
Data Usage | Uses historical data for training | Uses training data to create new data |
Task Type | Often used for regression and classification tasks | Commonly applied in content creation and generation |
Output | Provides predictions, forecasts, or classifications | Produces new content such as images, text, music, etc. |
Examples | Predicting stock prices, weather forecasting, customer churn | Creating paintings, music compositions, text generation |
Techniques | Utilizes machine learning algorithms for pattern recognition | Employs generative models like VAEs and GANs |
Approach | Focuses on learning patterns and correlations from data | Concentrates on generating new data based on learned patterns |
Data Requirement | Relies on historical data and labeled examples | Needs large amounts of unlabeled data for training |
Use Cases | Helps in decision-making and future planning | Fosters creativity and innovation in various domains |
Real-world applications of Predictive AI
Predictive AI and predictive analytics have been pioneering in the business world. As strategizing and forecasting demand is a big business KPI, a predictive type of AI becomes a valuable tool offering insights leading up to business growth.
Here are some applications and use cases to give you a better understanding of what is predictive AI.
1. Banking and Finance
Predictive AI became a transforming tool for the finance and banking sector in spotting fraudulent behavior and transactions. Predictive AI algorithms allowed these institutions to spot anomalies and suspicious behavior that could potentially be a sign of fraud.
The anomaly might be frequent switching of devices, login from a suspicious IP address, location, or purchase requests by a user that might seem unusual.
2. Marketing
Analyzing data to identify market trends and choosing the ideal marketing channel for them is a major activity that marketing entails. Predictive AI processes historical marketing records in seconds to generate insights that help curate a fool-proof marketing strategy backed by data.
Predictive AI can more closely define the most appropriate channels and messages to use in marketing. It can provide marketing strategists with the data they need to write impactful campaigns, bringing greater success.
3. Healthcare
Like generative AI, predictive AI is also hugely beneficial in healthcare. We all know that Covid19 hit the world like a sledgehammer. Almost every country was shut down for months, and medical infrastructure was shambling.
But what if we were able to predict it was coming? This is exactly what Predictive AI aims to resolve. The technology is already helping in predicting disease outbreaks, identifying patients with high risks, and forecasting seasonal inflation of certain flues. Moreover, Predictive AI opens the doors for personalized medicine. AI technology also helps customize treatment plans specific to a patient’s medical history.
Use of Generative AI in Business Operations
While Generative AI is used primarily by individuals to help in their everyday tasks, it also has a wide range of applications in the business sector. These applications span across various sectors, assisting in creating more innovative solutions and enhancing productivity.
Let's explore some key applications of Generative AI examples in different business sectors:
1. Manufacturing
Generative AI is already hitting a reset button in the manufacturing industry, simplifying and automating various human-intensive tasks with a flair of creativity. The technology utilizes various technologies to generate innovative designs and optimize manufacturing processes, producing efficient and effective production outcomes.
Mentioned below are some technologies that benefit from Generative AI in the manufacturing sector:
- 3D printing
- Computer-Aided Design (CAD)
- Machine Learning Algorithms
- Topology Optimization
- Finite Element Analysis
Let’s look at a real-world example, general electric, one of the leading aviation equipment manufacturers, opted for generative AI to create a lighter jet engine bracket. They fed constraints and requirements into the system and received an optimized design that reduced the weight of the bracket while maintaining its strength. The result? The new design was 75% lighter than the one created with AI input.
2. Healthcare
The one area where Generative AI is most promising is the healthcare and drug innovation sector. Typically, synthesizing new compounds for medical research is a labor-intensive task. It is a slow process as each experiment demands time and human intervention.
With generative AI, algorithms trained on large molecular datasets can propose drug candidates with similar properties to known drugs, potentially reducing the time and cost of developing new drugs.
The company Insilico Medicine was ahead of the curve and was using generative AI long before it caught the eyes of the mainstream media. The company leveraged generative AI in the preclinical drug discovery process.
As a result, Insilico Medicine successfully developed a potential drug for fibrosis using Generative AI. The company identified a molecule that a drug compound could target and could even predict the outcome of clinical trials with ML-infused Generative AI, which typically takes years using traditional methods.
3. Retail
The retail sector is also heavily invested in the AI race, among others. As observed by Precedence Research, the global market size of AI in retail was $8.41 billion in 2022. However, this number is predicted to rise to a staggering $45.74 billion by 2032, giving you a glimpse of the rising demand for AI in Retail.
Alibaba, a leading player in the retail and e-commerce space, has also dipped its toe into AI and predictive analytics. The company has amalgamated Generative AI and predictive analytics in its daily operations to cater to the need of millions of daily visitors. Alibaba uses natural language processing to generate product descriptions within seconds for the site, enabling faster and more efficient product listings.
Making the Final Call: Predictive AI vs Generative AI
Well, in the end, we can say that the rivalry between predictive AI vs generative AI tools should be looked at with a different lens. Both types of AI come from a single source. The only distinction remains in their goals and purposes.
Adopting these technologies solely depends on the requirements or the type of output you desire from the model. It is believed that as the capabilities of AI evolve, distinctions between these technologies will also dissolve.
However, in the present scenario, both types of AI offer groundbreaking value to businesses and individuals alike. Many companies also want to bump up their game with AI to gain that competitive edge. So, if you also want to integrate AI into your business, reaching the top Artificial Intelligence Companies might be a favorable choice.
We hope this article thoroughly examined Generative artificial intelligence vs predictive analytics for you and helped you better understand the difference between the two.